Honorable Opening Talk
Emeritus Prof. Christos Kitsos
Department of Informatics, University of West Attica, Greece
Invited Keynote Speakers
Prof. Mei-Ling Ting Lee
Department of Epidemiology and Biostatistics, University of Maryland, College Park, USA
Prof. Milan Stehlík
Institute of Statistics, University of Valparaíso, Chile
Prof. Valentina Escott-Price
Dementia Research Institute at Cardiff, Centre for Neuro-Psychiatric Genetics and Genomics, School of Medicine, Cardiff University, UK
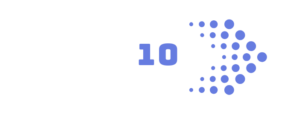
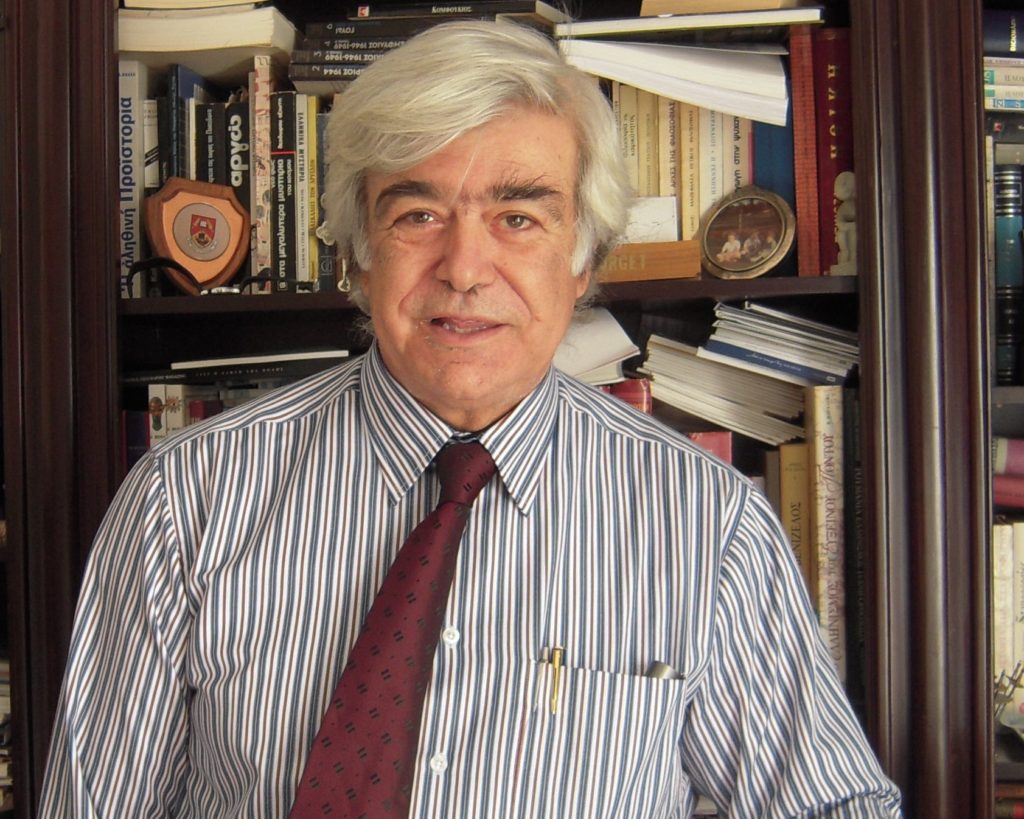
Department of Informatics, University of West Attica, Greece
Back to Top
Title: Extending and Strengthening the Risk Analysis Framework with ICRA
Abstract
The target of this paper is to summarize the extensions attempted the last 20 years, 2003-2023, through ICRA, and proposed more areas of interest. The Risk Analysis, although started for Political/Military oriented problems, moved to Biostatistics to offer qualitative methods for the appearance, mainly, of cancer. The “odds ratio” and the “unconditional logistic regression, were applied to a number of Ca problems. CYP3A isozymes are involved in tobacco carcinogen. Therefore studies of CYP3A4 and CYP3A5 genotype-dependent “risk for lung Cancer” are under consideration. In Cancer studies, more mathematics were involved, under Fractal Analysis, while the Relative Risk (RR) can be defined for the family of the γ-order Generalized Normal. The Environmental Risk appears to be a trivial extension adopting Birnbaum-Saunders distribution, or other generalizations of the Normal, while the identification of Pollution Level, needs the appropriate study.
Risk Analysis has been proved appropriate in studding Hedge Fund Investments, and Risk Management techniques, in some cases can be based on Tolerance regions, rather than Confidence regions. The strategic risk needs more extension we believe and the Patterns of Strategy for Risk Assessment and Management might be considered under qualitative methods.
Brief CV
Christos P. Kitsos was born in Athens, in 1951. He got his BSc degree in Math at the Univ. of Athens, in 1973, his MA degree in Math and Stat at Univ. of New Brunswick, Canada in 1978, and his PhD degree with his thesis on “ Design and Inference in Nonlinear Problems” at Pept of Stat., Univ. of Glasgow, UK on 1986. He worked as a Statistician at the early stage of his career in a number of jobs including the Greek Statistical Service, Greek Army, Athens Urban Transportation System, and Electrical Buses of Athens, and since 1986 he has devoted his activities to lecturing at Greek Universities.
He joined under various contracts the Greek Universities of Patras, Athens, Thessaly, Aegean, and AUEB, while he spent his activity as a Professor of Statistics at the former TEI of Athens, now University of West Attica for 30 years – he is an Em Prof. now. His research activities can be traced at Technometrics, Biometrics, IEEE, Comm. in Stat., REVSTAT among others, as well as in proceedings volumes with a total of more than 180 today (Dec24), with more than 1100 citations.
He is co-editor of 8 international volumes (Physica-Verlag, Springer, Wiley), a number of Greek volumes, and 8 textbooks for Statistics in Greek. His research interests concern, Optimal Experimental Designs, Information measures, Industrial Statistics, Environmental problems, Tolerance Regions, Cancer Bioassays, gamma-order generalized distributions etc.
He has run ICRA since 2003, he served as a Head of the ISI, Committee of Risk Analysis, and worked as an editor to Springer volumes of ICRA.
Back to Top
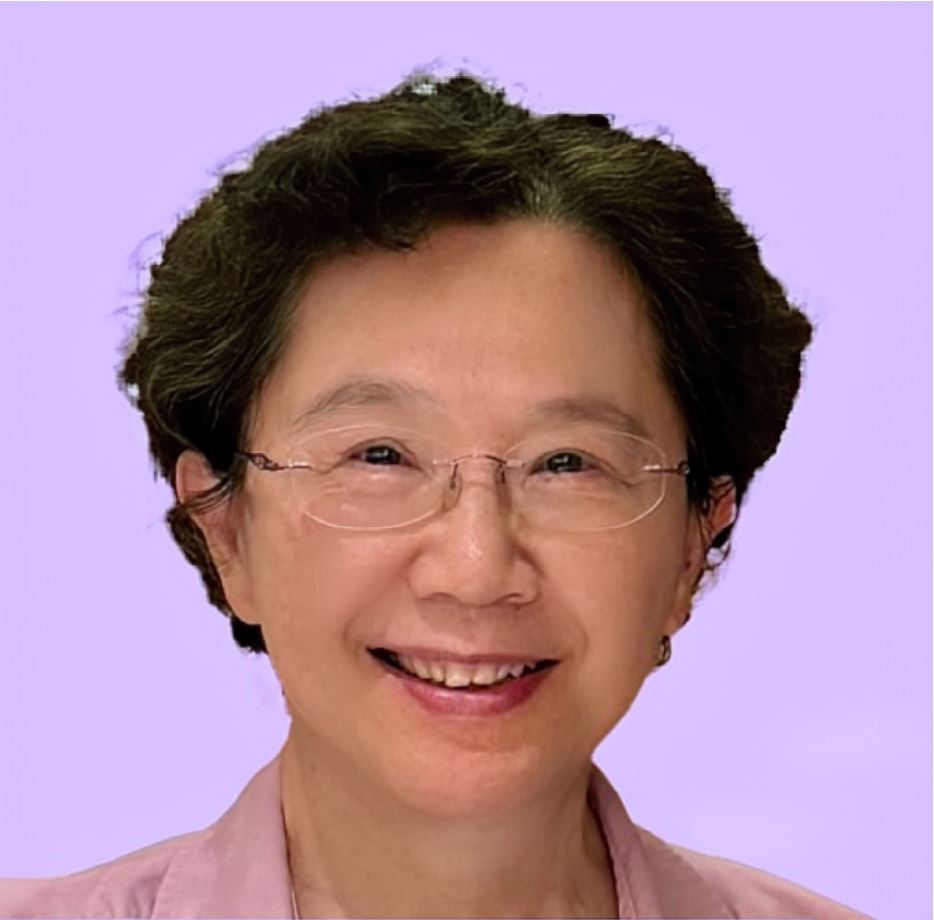
Title: Stochastic First-hitting-time Models with Extension to Neural Networks
Abstract
Neural networks are a subset of artificial intelligence (AI). I’ll give a brief introduction of the concepts and then explain how to use it.
Degradation of an engineering system or a patient’s health can be described mathematically by a stochastic process. The system (or the patient) experiences a failure event when the condition of the system (or the patient’s health) decreases to a critical event threshold level. This happening defines a failure event and a first hitting time (FHT). First hitting time risk models are based on an underlying stochastic process; they do not require the proportional hazards assumption and represent a realistic alternative to the Cox model. I’ll present the methods to extend the FHT risk models to neural networks.
Brief CV
Dr. Mei-Ling Ting Lee is a Professor in the Department of Epidemiology & Biostatistics at the University of Maryland, College Park. She developed a statistical model called the first hitting-time based threshold regression (TR) for analyzing time-to-event survival data. The first hitting-time TR model has been extended to machine learning neural networks for AI applications. Dr. Lee is the Founding Editor and Editor-in-Chief of the international journal, Lifetime Data Analysis, the only journal with an emphasis on methods for analyzing time-to-event data. The journal is currently publishing the 30th volume. Dr. Lee has published more than 150 articles including bioinformatic methods for high-dimensional genomic data. She published a single-authored monograph titled “Analysis of Microarray Gene Expression Data” in 2004. Dr. Lee also co-edited three other books.
Back to Top
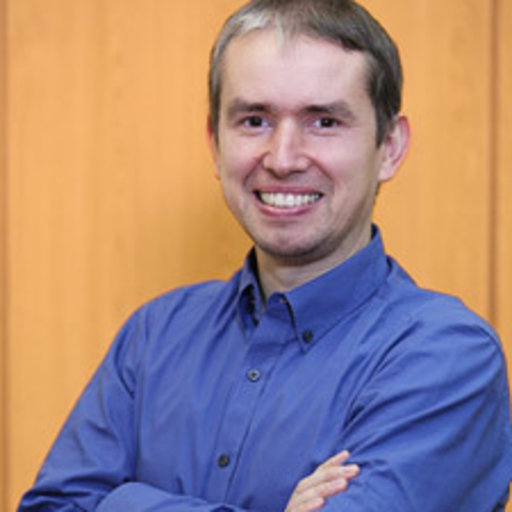
Back to Top
Title: Advanced classifications and analyses of bacterial growth and biofilm production of Cobetia sp. MM1IDA2H-1: from fractional to hybrid systems
Abstract
Biofilms are complex biological structures built by microorganisms with biomolecules over solid or liquid surfaces, producing significant problems in health, food safety, and maritime infrastructure (ports and ships). Using the super biofilm producer Cobetia marina strain MM1IDA2H-1 as a model growing in a wide range of temperatures, our aim is to provide statistical support for biological hypotheses on biofilm evaluations across wide ranges of temperatures where bacteria can grow with phenotypical variability such as biofilm behavior. Biofilms were measured across a wide range of growth temperatures at the exponential growth phases. A non-standard ANOVA-like approach to assessing heterogeneity across temperatures is developed to explain biological behaviors related to biofilm formation, biomass, and temperatures. An exponential family-based ANOVA-like analysis with a novel statistical approach has been developed, providing deep biological insights. When considering growth curve classifiers, the standard numerical derivative has not worked well in discrimination, so we need to develop a novel approach, such as fractional derivatives, and implement it. Since the biofilm variable is defined as a ratio of generally two non-unimodal random variables, important statistical issues should be addressed in order to create precise classifications with good properties. False positivity should be maintained in the tractable range. This motivates the construction of a hybrid system, where a deep neural network can be implemented. In particular, in one of the hidden layers, the SPOCU transfer function is found to be a good choice.
Brief CV: To be announced
Prof. Milan Stehlík is a Professor of Statistics at the Institute of Statistics, University of Valparaíso, Chile, and previously served as Associate Professor at Johannes Kepler University (JKU) Linz, Austria. His research spans statistical modelling in medicine, finance, and environmental science, with a strong focus on multi-criteria decision-making under uncertainty, stochastic dependence, and the development of robust methods for analysing complex data. Prof. Stehlík has collaborated with institutions such as Oxford, Stanford, and UCLA, and has led interdisciplinary projects on topics ranging from cancer diagnostics to modelling glacier changes in Chile.
In addition to his research, Prof. Stehlík plays a significant editorial role in the academic community. He serves as Editor-in-Chief of Research in Statistics and as Associate Editor for both Neural Computing and Applications and the Journal of Applied Statistics. He has organised several international workshops and delivered keynote lectures worldwide, including recent events in Chile, Portugal, and Austria.
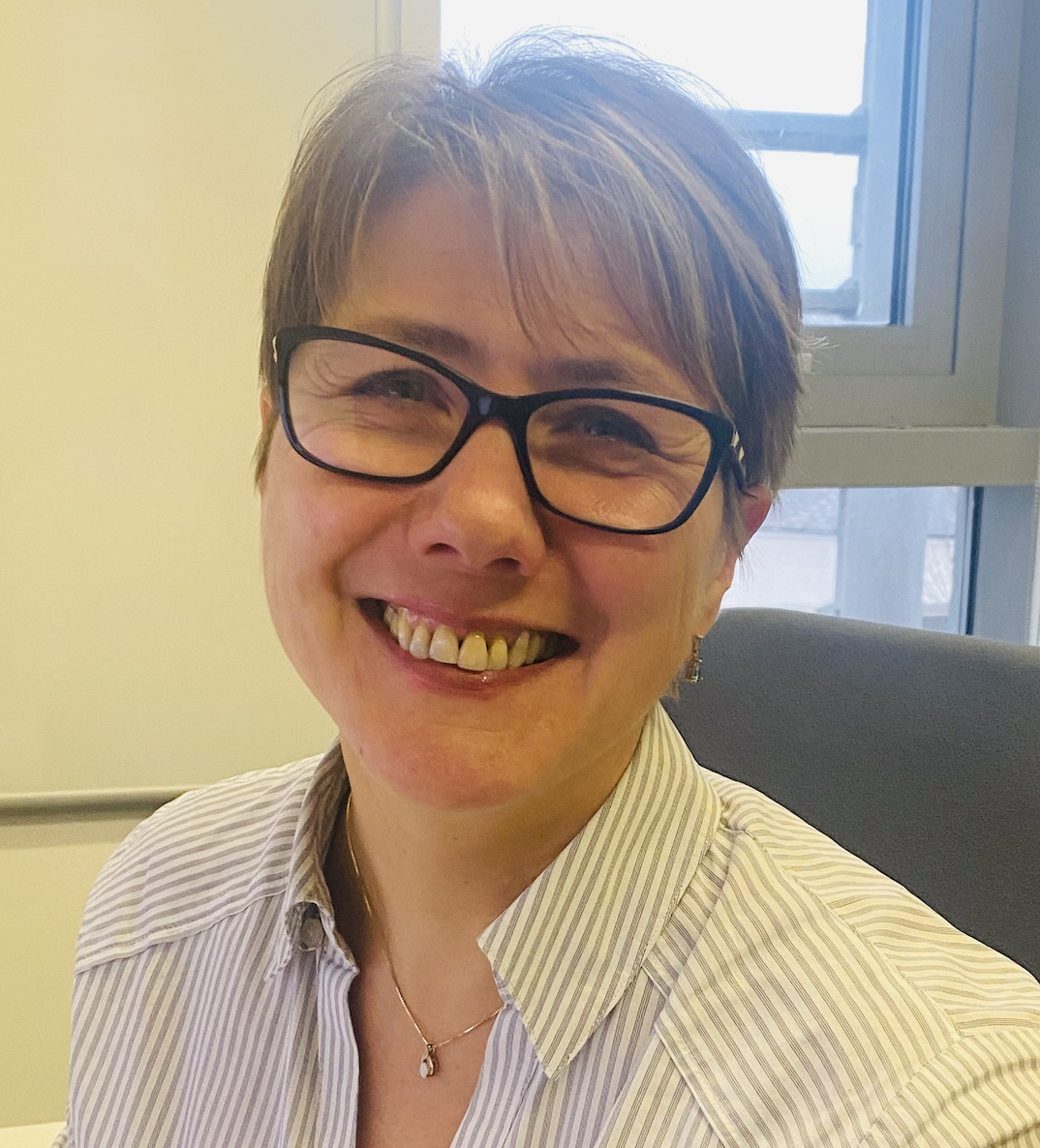
Dementia Research Institute at Cardiff, Centre for Neuro-Psychiatric Genetics and Genomics, School of Medicine, Cardiff University, UK
Back to Top
Title: Genetic Risk Prediction for Brain Disorders: Current Insights and Future Directions
Abstract
Brain disorders are complex and often highly heritable traits that can be caused by a combination of genetic, physical, psychological and environmental factors. Such complexity is evident in their diagnosis, which is often based on symptoms. Differentiation between brain disorders is further challenged by a pronounced overlap in symptoms and comorbidities. Neurodegenerative disorders, like Alzheimer’s disease, triggers a range of psychiatric symptoms, including depression and anxiety, in addition to physical difficulties like incontinence. The phenotypic complexity of brain disorders is mirrored in their genetics.
Polygenic risk scores are a method increasingly used to capture the combined effect of genome-wide significant variants and those which individually do not show genome-wide significant association but are likely to contribute to the risk of developing diseases. However, their practical use incurs complications and inconsistencies that so far limit their clinical applicability.
With expanding datasets and computational advances, Machine Learning (ML) has the potential to further enhance risk prediction and gene discovery, particularly through federated learning and multi-omic integration. A key challenge is the ability to condense and filter large-scale data, effectively distinguishing signal from noise while capturing the complexity of brain disorders. The success of ML largely depends on data quality, the features extracted, the choice of objective or loss function, and the selection of an appropriate model architecture and hyperparameters that best fit the research question.
Future advancements will rely on the willingness of researchers from non-computational disciplines to collaborate openly with mathematicians and computer scientists, their readiness to share data, and a collective effort to carefully develop, interpret, and report results.
Brief CV
Valentina Escott-Price is a Professor in Biostatistics at Cardiff University, specializing in statistical modeling, genetic epidemiology, and machine learning applications in neurodegenerative diseases. As a leading researcher at the Dementia Research Institute (DRI) at Cardiff, she has played a key role in advancing genetic risk prediction for Alzheimer’s disease, demonstrating its polygenic nature and its potential for clinical applications. Her expertise in analyzing large-scale genetic and clinical datasets has contributed to groundbreaking insights into the genetic mechanisms underlying psychiatric and neurodegenerative disorders.
With over 300 publications, including research in Nature, Science, and Brain, she continues to push the boundaries of computational approaches in biomedical research. Her recent work integrates artificial intelligence and federated learning to enhance disease risk prediction and patient stratification using national and international health data resources. By developing novel statistical methodologies and leveraging big data analytics, she aims to revolutionize personalized medicine approaches for dementia and related diseases.
Full CV
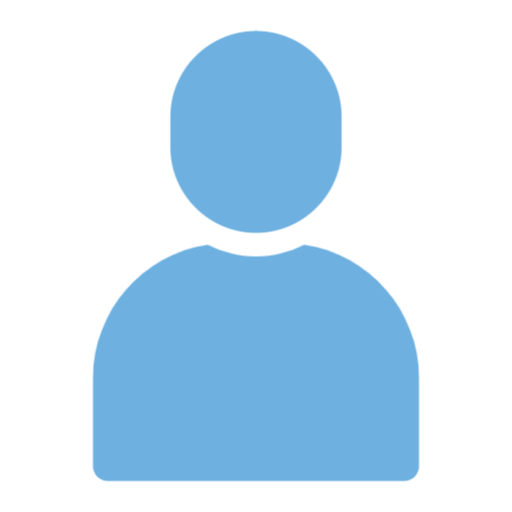
To be announced
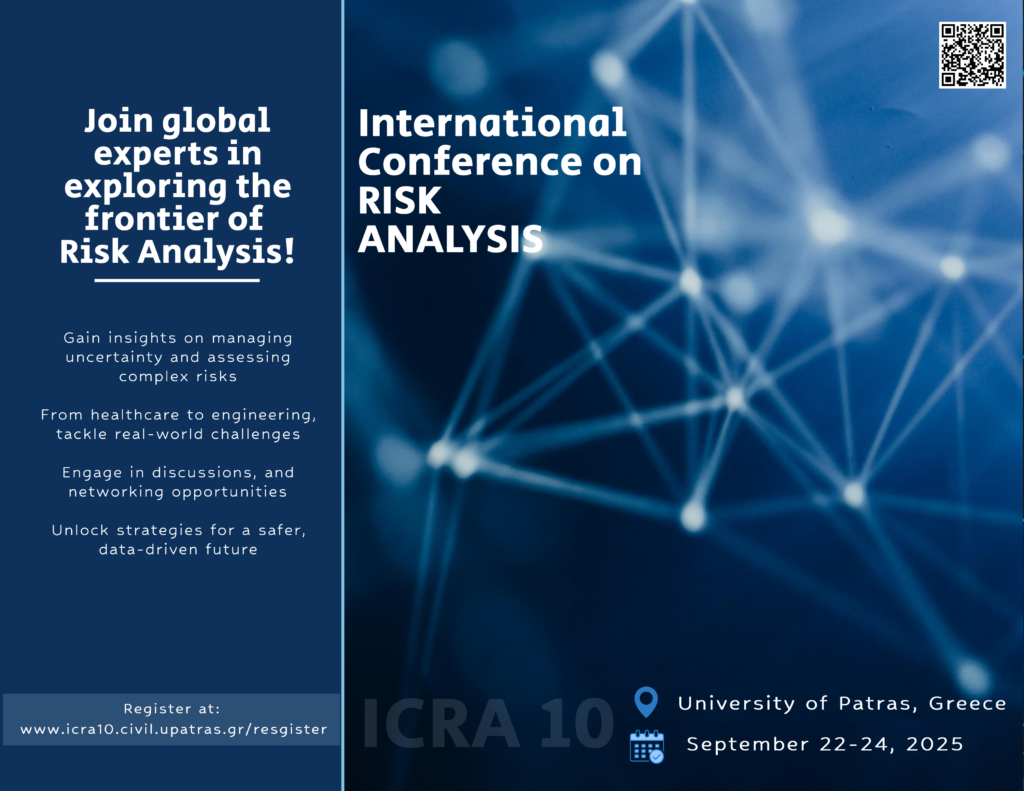